Traders are constantly on the lookout for opportunities to generate profits in the dynamic world of finance . One approach that has gained popularity is statistical arbitrage which is also known as stat arb . This trading strategy relies on mean reversion analysis and involves investing in diverse portfolios of securities for short periods of time which range from a few seconds to multiple days .
Stat arb is a highly quantitative and analytical approach to trading . It aims to minimize exposure to market instability by employing two key phases: “scoring” and “risk reduction .” In the scoring phase stocks are ranked based on their desirability as investments . The risk reduction phase then combines selected stocks into a portfolio designed to minimize risk . Traders typically use mathematical modeling techniques to identify arbitrage opportunities .
The Market Neutrality of Statistical Arbitrage
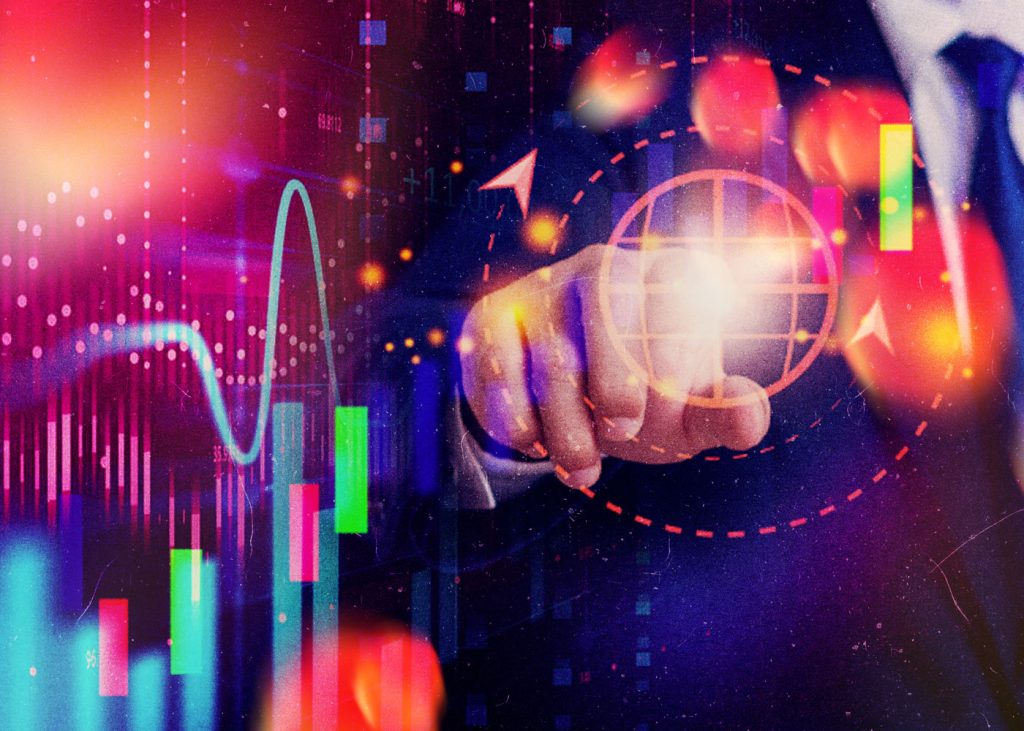
One distinguishing feature of statistical arbitrage strategies is their market neutrality . This means that traders open both long and short positions simultaneously to capitalize on pricing inefficiencies in correlated securities . For instance if a fund manager believes that Coca-Cola is undervalued compared to Pepsi they would open a long position in Coca-Cola while simultaneously opening a short position in Pepsi . Traders often refer to this method as “pairs trading .”
Statistical arbitrage is not limited to just two securities . It can be applied to a group of correlated securities . What’s more is that correlation can exist between stocks from different industries . For instance, Citigroup which is a banking stock and Harley Davidson which is a consumer cyclical stock can exhibit periods of high correlation .
Risks and Opportunities in Statistical Arbitrage
While statistical arbitrage can be a profitable strategy it is not without risks . Its success centers on market prices reverting to historical or predicted norms which is a concept known as mean reversion . However two stocks that operate in the same industry can remain uncorrelated for extended periods of time due to various micro and macro factors .
Many statistical arbitrage strategies rely on high-frequency trading (HFT) algorithms to capitalize on small pricing inefficiencies . These algorithms exploit brief opportunities that last for milliseconds . However this approach requires large positions in both stocks to generate meaningful profits from minuscule price movements which introduces additional risk . Options can be used to lessen some of this risk .
Getting started with statistical arbitrage doesn’t necessarily demand advanced math skills . Traders can begin by identifying two traditionally correlated securities such as General Motors (GM) and Ford Motor Company (F) and comparing their price charts . By entering trades when the stocks significantly deviate from each other traders can aim to profit when the prices eventually realign . However it’s important to note that there is no guarantee of when or if the prices will converge so implementing stop-loss orders is advisable .
Final Thoughts
Statistical arbitrage offers traders a modern approach to generating market neutral profits . By leveraging mean reversion analysis and pairs trading strategies traders can capitalize on pricing inefficiencies in correlated securities . While the strategy carries inherent risks such as the reliance on mean reversion and the need for high-frequency trading it can be a beneficial option when it is executed with careful consideration and risk management .