Data science and the principles of analytics have become pivotal within the field of credit risk data science, allowing for more efficient methods for creating accurate credit profiles. Used increasingly across every sector, from individual consumers to large corporations and investment banks, data science is now a fundamental cornerstone of modern financial systems. By combining cutting-edge technological tools with mathematical algorithms, organizations can gain deeper insights into customer patterns and develop strategies based on accurate findings to reduce their overall credit risk exposure while maximizing valuable opportunities. This blog post will examine how data science has transformed credit risk management.
What Is Data Science?
Data science is an interdisciplinary field involving the extraction, analysis, and interpretation of complex data using statistical and computational techniques. It’s a rapidly evolving field that’s getting more attention with the advent of big data, machine learning, and artificial intelligence.
Data science involves various technologies and tools, including statistical methods, machine learning algorithms, programming languages, and data visualization techniques. Data scientists can use these tools to extract meaningful insights from large and complex data sets that they can use to make decisions, improve business operations, and gain competitive advantage.
The data science process typically involves multiple phases, such as data collection, preparation, analysis, and interpretation. The first stage collects and stores data from various sources, including structured and unstructured data. Data preparation involves cleaning and preprocessing the data to remove errors and discrepancies and prepare it for analysis.
Data analysis involves using statistical and machine learning techniques to extract patterns and trends from data. This includes techniques such as regression analysis, clustering, classification, and neural networks. Data visualization is an essential aspect of data science, presenting data in visual formats such as charts, graphs, and heatmaps to make it easier to understand and interpret. Data science has many applications across industries, such as healthcare, finance, marketing, and manufacturing. For example, in healthcare, data science can analyze patient data, identify disease risk factors, and create personalized treatment plans. In finance, data science can research financial data, predict stock prices, identify investment opportunities, and uncover fraud.
In summary, data science is an interdisciplinary field that uses statistical and computational techniques to extract insights from complex data sets. It is a rapidly developing field with numerous applications in various industries and is expected to grow in importance as the amount of data continues to grow.
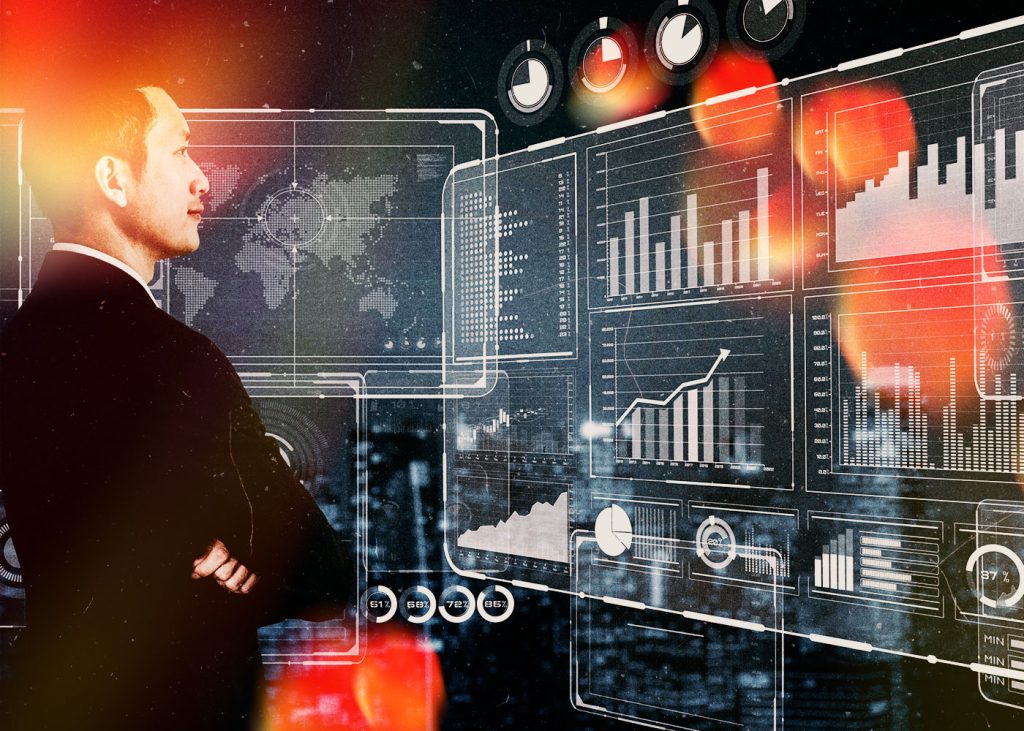
What Is Credit Risk?
Credit risk is the possibility that the borrower will default and the lender will suffer losses. In other words, it is the risk that the borrower will default on the loan or obligation, which could result in financial loss for the lender.
Credit risk arises when lenders, such as banks and financial institutions, extend credit to borrowers, such as individuals and businesses, based on their ability to repay the loan. A borrower’s ability to repay a loan is typically determined by assessing their creditworthiness, which includes factors such as credit history, income, employment status, and other financial metrics.
Credit risk can be categorized into two types:
- Individual credit risk and systemic credit risk. Individual credit risk is the risk that a particular borrower will default, while systemic credit risk is the risk that a large number of defaults or major economic events could impact the financial system as a whole. .
- Lenders use a variety of methods to manage and mitigate credit risk. One such method is credit scoring. It uses statistical models to assess a borrower’s creditworthiness and assign a credit score. Lenders also use collateral or collateral, such as real estate and assets, to mitigate credit risk.
Additionally, lenders can diversify their loan portfolios by offering loans to different borrowers in different industries and geographies to spread risk. In summary, credit risk is the inherent risk associated with lending and borrowing. If the borrower cannot repay the loan, the lender risks losing money. Credit risk management is therefore important for lenders to minimize financial losses and ensure the stability of the financial system.
What Is the History of Credit Score?
The roots of modern credit scoring were laid by Ronald A. Fisher, who in 1936 provided the statistical method of discriminant analysis. This approach was applied to distinguish groups based on a list of measurable attributes. In 1941, Durand discovered that this method could be used to determine good or bad credit.
So, as the name “credit scoring” suggests, financial institutions assign applicants several credit scores. These scores serve as a numerical representation of the probability that a loan applicant will repay the loan in full on time. Banks use credit scores for risk-based pricing. In other words, borrowers with lower credit scores are more likely to get loans with higher interest rates and worse terms.
The biggest problem with the traditional (scorecard-based) approach to credit evaluation is its rigidity. Because the conventional system is rule-based, individuals are less likely to get a new loan from a banking institution without a complete track record of previous loans.
But how does an unbanked person get their first loan? And how can someone looking for money to start a business get the money to pay off a loan? These subtleties are not included in traditional approaches to credit modeling. Only bankable people with a good history of credit services can get more credit in the future.
Based on current estimates, the main deficiencies that prevent traditional credit modeling systems from providing accurate credit ratings are:
- Analysis based only on historical data
- Consideration of variables in a vacuum.
- Human biases and perceptions falsify risk assessment parameters
- Ability to evaluate linear relationships only
- Based only on rule-based logic, not subtle
- Improved reliability of structured data
Data science can be applied to financial services in many ways. The first step is understanding the data and performing feature engineering on the base model. Based on this, you can later customize the ML model to serve your credit scoring use case.
What Is Credit Risk Data Science?
Credit risk data science analyzes and manages credit risk using data science techniques. Credit risk refers to borrowers’ likelihood of defaulting on their loan or credit card payments. To order this risk, financial institutions use various data science techniques to analyze data about borrowers’ credit histories, financial statements, and other relevant information.
Credit risk data science uses statistical models, machine learning algorithms, and data visualization tools to analyze data about borrower behavior and credit risk factors. This includes analyzing credit scores, payment history, income, debt-to-income ratios, and other factors affecting a borrower’s creditworthiness. Data scientists in credit risk management also use predictive modeling techniques to predict the probability of default and identify riskier borrowers. This allows financial institutions to take appropriate steps, such as: Adjusting interest rates, lowering credit limits, or denying credit to riskier borrowers
In addition to analyzing borrower data, credit risk data science also involves monitoring and analyzing market trends and economic indicators that can affect credit risk. With these factors in mind, financial institutions can make informed decisions about their lending practices and adjust their risk management strategies accordingly.
Overall, credit risk data science plays a crucial role in managing credit risk and ensuring the financial stability of financial institutions that offer credit products.
Summarize
Credit risk data science has become increasingly important with the rise of global economic interconnectivity. With the right analytical tools and techniques, such as those made available through data science, organizations can develop effective strategies to understand better, mitigate, and manage risk associated with credit products and services.Whether you’re an executive at a financial institution or an individual looking to protect your assets, recognizing current trends in credit risk data science is essential for finding success in today’s economy.